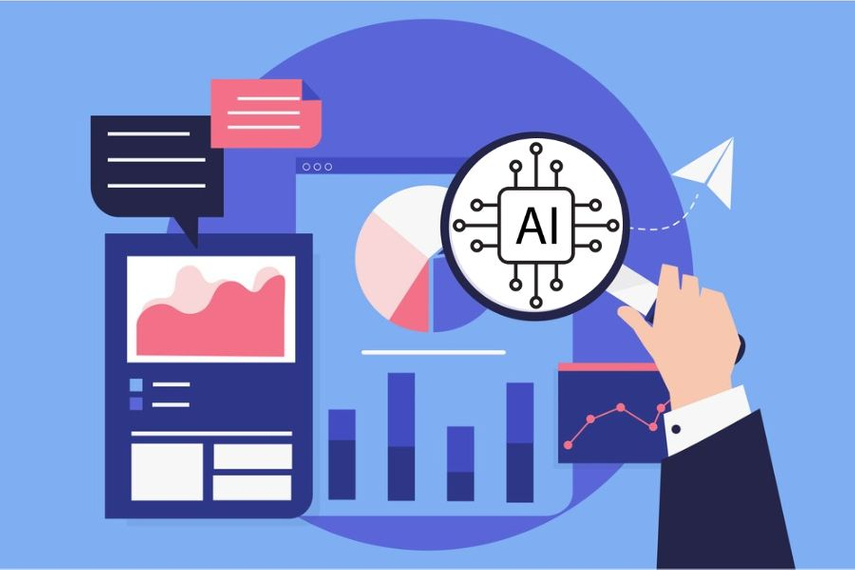
This article is being written at the right time, or probably a little late, given the speed with which conversation, use cases, and perspectives have exploded in the recent past. Ever since the success of Chat GPT’s generative AI platform; the steadily growing usage of AI in research and data sciences has transformed into a steep growth curve, with GenAI emerging as the new buzzword.
Why this topic? Why now?
AI in general, and GenAI in particular, has the potential to grant enormous power to market research and data science practitioners and end-users alike—manifested in terms of huge capital and time savings. In some cases, it can even lead to downsizing headcount. However, this power is often unsupervised or ‘unlicensed’. Imagine giving the keys of a powerful sports car to a teenager without a driving license, or scalpels and surgical knives to a medical student to perform a surgery without due supervised training on cadavers.
One may argue that the said license in our field has less potential for damage as it doesn’t take lives. But consider the millions that may go down the drain with incorrect business decisions and incorrect judgment of human potential in the workplace.
AI in research and data science
Initially, AI in research focused on summarising and analysing data with human interpretation. Human intelligence was crucial for and integral to programming and interpreting AI results during the early adoption phase. The development of Large Language Models (LLMs) has led to the creation of generative AI platforms. Generative AI's training involves diverse datasets from books, reviews, articles, and social media to ensure broad applicability. Unlike earlier AI models, GenAI can independently generate insights across topics, broadening its applicability across fields and enhancing business strategies.
While this generalist approach gives these platforms inclusive and eclectic reach, it is also key to watch-out when it comes to domain-specific use cases. Needless to say, market research and data science-based insight and consultancy land us in the domain of knowledge-based specificity. Given this context, let’s examine the key learnings that allow us to harness the potential of AI while mitigating the risks of its unethical or irresponsible usage.
Championing ethical and responsible AI
To understand this better, let’s consider what irresponsible and unethical AI may constitute. The ubiquitous reach of ‘off-the-shelf’ GenAI models leads to its potential unethical uses. Use of confidential data or data obtained for specific reasons from participants or clients to train GenAI models for more general uses; constitutes its unethical use. Transparency and integrity around the source of training data, informing the source about ways and means that data can be used—which now includes training GenAI models—need to be ensured. This responsibility rests primarily with us, the community of market research and data science practitioners.
Responsible GenAI use is in the specificity of the domain and relevance of use cases. For example, if one uses a GenAI model trained on consumer reviews obtained from developed markets like the US and Western Europe to predict responses to a new car launch in India, there will be a significant chance that the model predictions will be inaccurate.
The responsible use of GenAI will be to train the model on data and reviews from Indian consumers. This may not be as easily and amply available as reviews from developed markets. But it is exactly that pain in terms of additional investments or time that constitutes our responsibility in this context. It means that we must have the right human experts who control the training of the model with the right datasets and review the outputs generated by AI. Responsible AI is likely to be more impactful AI, ensuring that your AI is unbiased and does not hallucinate.
A best practice framework
Let's explore a best practice framework that involves ensuring confidentiality and compliance by treating participant and client data with security, fairness and integrity. Here, the well-trained and experienced human is in the loop. While AI answers the questions, the human intelligence (HI) questions the answers.
Over the years, the insights and data science organisations have made investments in domain-specific expertise and learning. We must integrate this rich learning into the power of AI to get the best impact. GenAI needs to be provided with grounding (read: training) in fresh, relevant, and real datasets. For example, training models based on Indian contextual data and writing prompts that are sensitive to local realities will go a long way in ensuring that your GenAI practice will be impactful and not just efficient. Do your AI use cases and practices follow the practices mentioned here?
AI and synthetic data
Let’s now discuss a few good practices to follow when it comes to use of synthetic data for AI training. Synthetic datasets deliver time and cost savings but also come with some risks. Synthetic data refers to artificially generated datasets that do not correspond to actual events, observations, or people’s responses. It can be useful only when it mimics the characteristics of an actual dataset in terms of statistics like means, dispersion, etc.
Common synthetic datasets, useful especially for large or complex surveys, include imputation, where missing values are replaced to complete partial datasets. Another practice, complete generation, entails replacing real data with synthetic options, which, however, may be fraught with data accuracy risks. A balanced approach is partial generation, where real data from a small sample is used to create a larger, representative synthetic dataset, to better reflect the characteristics of the original sample.
Although not as widely adopted as GenAI, synthetic data demands cautious optimism. At Ipsos, we found that methods like Generative Adversarial Networks (GANs), though older, are more effective but still require expert supervision. Datasets must be tailored to specific domains, such as product testing or usage studies, with validation from human experts. The quality of synthetic data depends on 'seeding' it with fresh, real data to ensure it mimics the original dataset’s statistical characteristics.
Implications for research and data science
One of my learned colleagues says that AI has never been a consumer, nor a market researcher, and definitely not a client. Human sensibilities and intuition cannot be replaced by a machine. Hence it’s important for humans to question the machine and re-iterate and re-train it to keep refining results.
Training AI using current and contextual data is necessary in India. Most off-the-shelf AI models have been trained on data from the western, developed world. That makes it imperative for us to bring fresh, contextual perspectives to the machine. We must also invest in building human resource capabilities at the intersection of domain and AI expertise.
Pure ML algorithms based on past data may be cheaper, but bringing fresh human data and local human expertise will go a long way in ensuring impact and guarantee better ROI. Similarly, synthetic datasets augmented with small samples of fresh data always work better than complete machine generated synthetic samples.
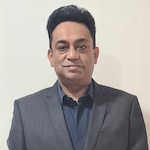
– Krishnendu Dutta, group service line leader – innovation, MSU, and strategy3, Ipsos India